Revolutionising Energy Storage: The AI and Experimental Design Fusion in Battery Tech
Heard lots of chatter around AI and Machine Learning but not entirely sure how it affects the battery industry? Well, this week we’ve teamed up with machine learning innovators Intellegens to bring you an introduction to how machine learning is supercharging battery optimisation.
Dr. Joel Strickland is a Principal Machine Learning Scientist at Intellegens with extensive experience in creating and applying algorithms to solve complex challenges in various industries. Joel focuses on practical AI applications and data analytics. He is committed to developing innovative, sustainable energy solutions through rigorous analysis and machine learning expertise.
Intellegens applies advanced machine learning to accelerate innovation for materials R&D. The Alchemite™ software enables you to extract maximum value from real-world experimental, process, and other data. Customers optimise products and processes, and save time and cost by achieving R&D objectives while reducing experimental workloads by up to 90%. The Alchemite™ method works for sparse, noisy data where other machine learning approaches fail. There have been successful applications across a wide range of materials-related processes in the battery space (https://intellegens.com/applications/batteries/).
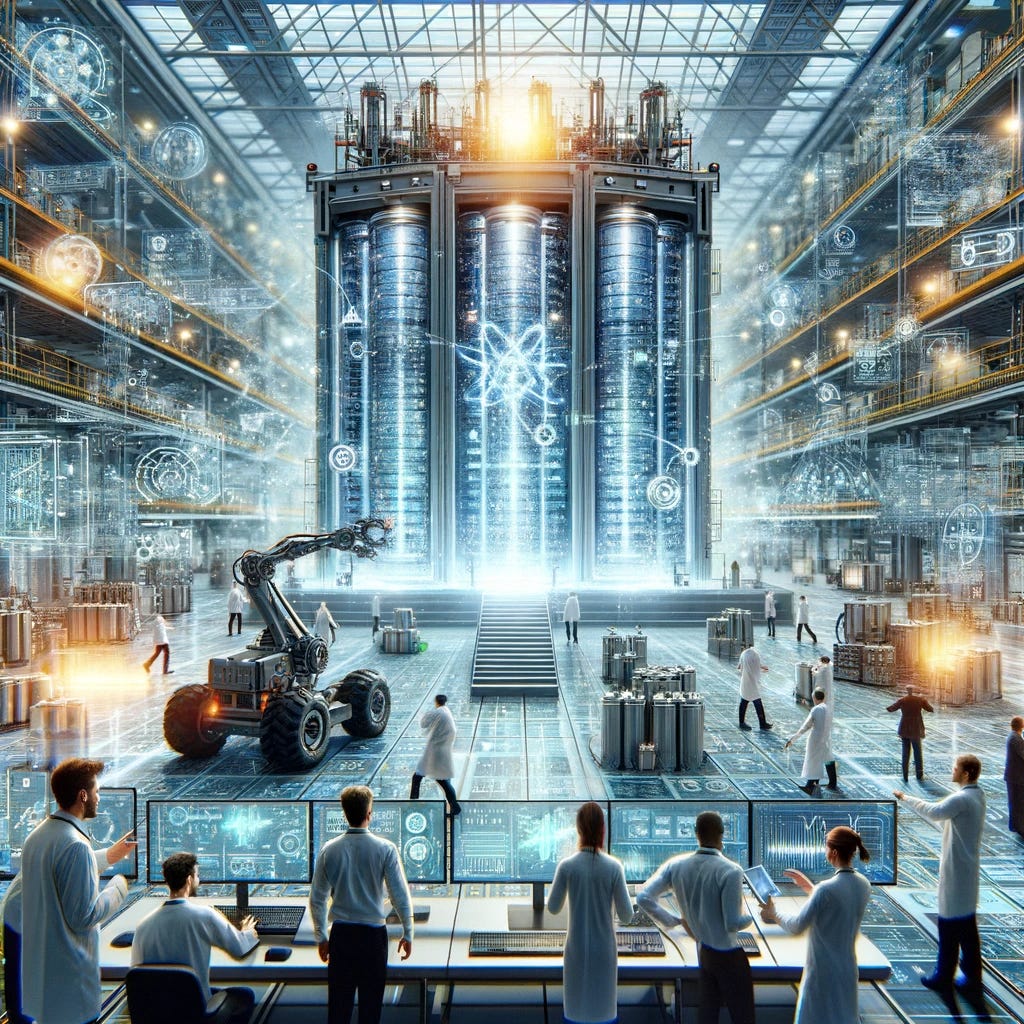
Introduction: The Current Landscape of Battery Technology
In a world constrained by electric vehicles with limited range, renewable energy systems struggling with storage efficiency, and mobile devices in constant need of recharging, the urgency for advanced battery technology has never been greater. Traditional battery research methods are reaching their limits, highlighting the need for a transformative change.
The pressing question is: how can we expedite innovation in battery research, and what impact will this have on our daily lives and the future of renewable energy?
The Dawn of a New Era in Battery Technology
The landscape of battery research is undergoing a transformative shift with the integration of machine learning into the adaptive experimental design methodology [1]. This marks a notable departure from conventional design of experiment approaches, paving the way for more innovative, efficient, and sustainable battery development [2]. The key question is: how do machine learning and adaptive experimental design juxtapose to revolutionise battery technology?
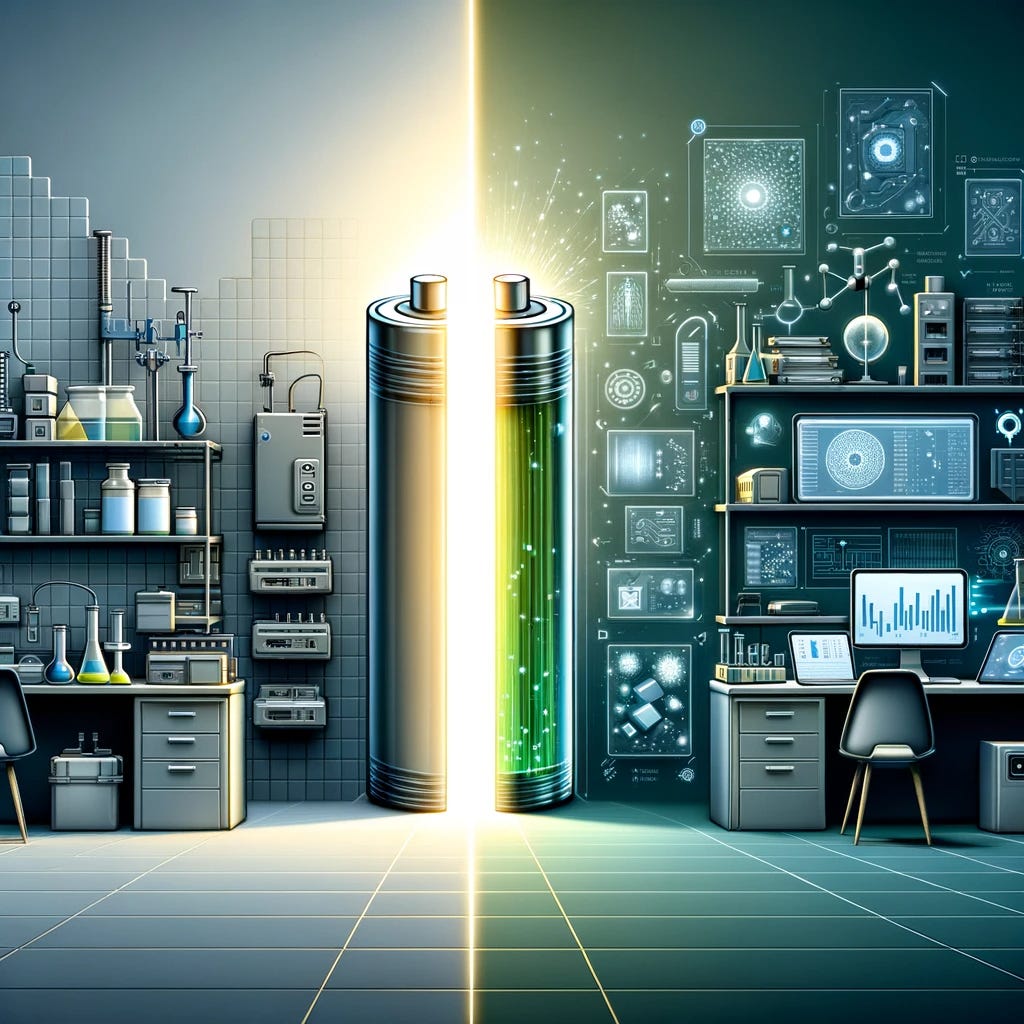
Section 1: Harnessing Data-Driven Machine Learning for Advanced Battery Design
Machine learning, a pivotal component of artificial intelligence (AI), is rapidly advancing battery technology research and development. It is significantly improving the efficiency, sustainability, and cost-effectiveness of design and manufacturing processes [3]. These sophisticated algorithms analyse various inputs to predict battery performance.
Importantly, effective machine learning models can work with datasets of various sizes. While comprehensive datasets are ideal, these models are also capable of extracting valuable insights from smaller, less complete datasets [4-6], a particularly pertinent factor in battery research, as illustrated below.
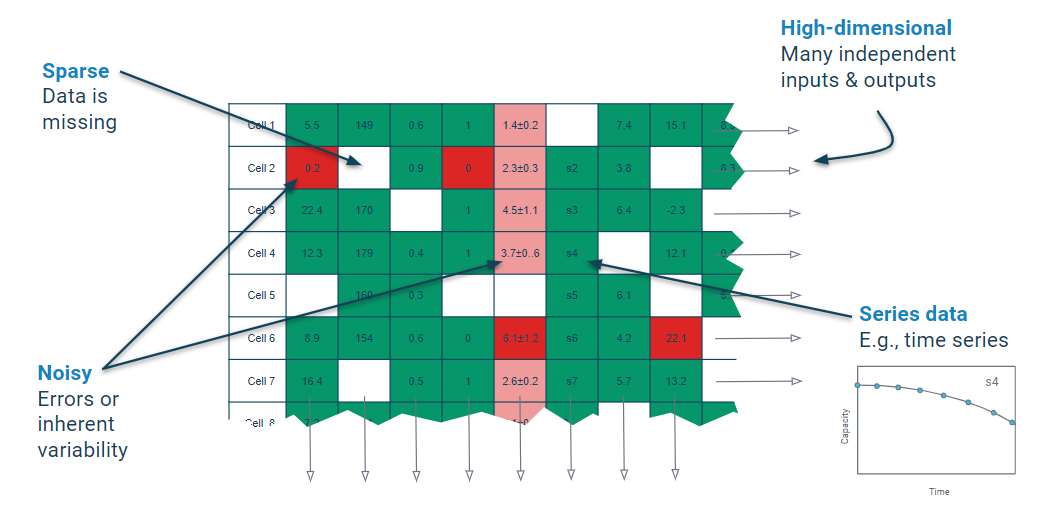
Impact in the Consumer Electronics Industry
In the consumer electronics industry, machine learning has already had a notable impact. Major smartphone manufacturers exploit machine learning to optimise battery life, geometry, and chemistry. Electric vehicle manufacturers also use algorithms that predict user behaviour and adjust power usage to prolong battery life, showcasing the practical benefits of machine learning.
The rapid development of this technology has been so remarkable that it has left many to marvel at how machine learning and AI have made such advancements possible.
Developing Machine Learning Models for Battery Performance
The development of machine learning models for battery performance begins with the collection of relevant data, a crucial first step as the quality and relevance of data directly impact the model's effectiveness.
Data Collection: Identifying Relevant Parameters
The process starts with gathering a dataset. For understanding and predicting battery performance, data can come from various sources, including experimental results, user usage logs, and real-world performance testing. This data should encompass information about processing conditions, design specifications, and battery properties. For example, in studying a smartwatch battery's performance, data might include the physical properties of the battery, usage patterns, and charging habits.
Feature Selection: Determining Key Predictors
Once a dataset is in place, the next step is identifying key features that significantly influence battery performance. This process involves selecting parameters most relevant to the aspect of battery performance being analysed, such as charging cycles for lifespan predictions or energy discharge rates for performance efficiency.
Model Construction: Choosing the Right Algorithm
Selecting the right features, such as usage behaviour, environmental conditions, and charging patterns, is crucial, especially for applications like a smartwatch battery where predicting time until recharge is required. These features should be fed into an appropriately chosen machine learning model.
Different models, such as neural networks for complex pattern recognition or regression models for more straightforward predictions, can be employed depending on the nature of the prediction task and the characteristics of the data.
Application and Continuous Improvement
These models enable advancements in battery technology, making devices like smartwatches more efficient and longer-lasting. In a competitive market, the continuous refinement of these models is key. Businesses analyse the alignment of their predictions with actual battery performance, making adjustments to improve accuracy and reliability. This ongoing process reflects a commitment to leveraging cutting-edge technology for enhancing battery performance and identifying new opportunities for innovation.
Section 2: Revolutionising Battery Research: Combining Adaptive Experimental Design with Machine Learning
Adaptive experimental design is a methodology where the experimental strategy evolves in response to preceding outcomes. This approach enhances the efficiency and focus of research. In the automotive industry, for instance, adaptive experimental design accelerates the development of more efficient batteries for electric vehicles (EVs) [3]. This strategy has been instrumental in producing batteries that not only charge more quickly but also have a longer useful life. Such advancements directly address key issues in the adoption of EVs, making them more appealing to consumers.
Combining Machine Learning for Smarter Battery Development
Combining machine learning with adaptive experimental design marks a significant advance over traditional design of experiment. This methodology excels at handling complex, multi-dimensional data (Fig. 1, above) to draw insights about battery performance, longevity, and the optimal charging procedure. Through a continuous process of learning and adjusting (Fig. 2, below), this approach guides crucial aspects of battery design and production, leading to the creation of batteries that are not only more effective but also more reliable.
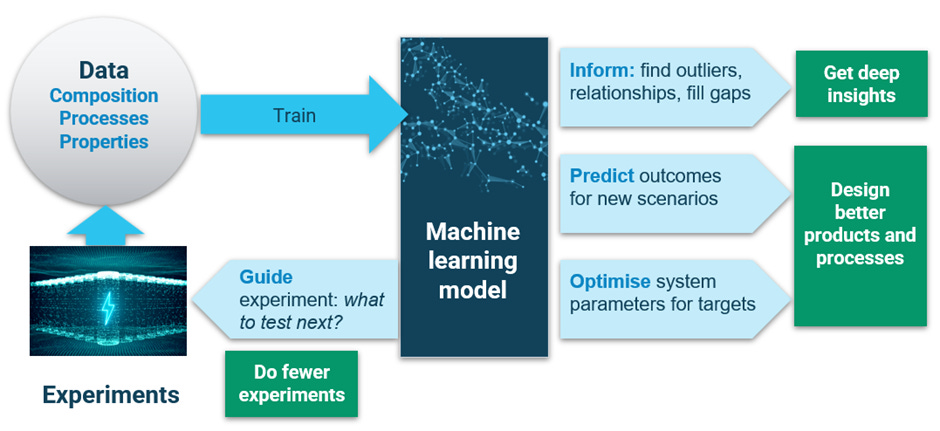
Streamlining Research for Greater Impact
Adaptive experimental design, now advanced with machine learning, sharpens the focus of research efforts on the most impactful areas. Researchers utilise advanced predictive models to more accurately select experiments with the highest potential for meaningful results. These machine learning models excel in evaluating uncertainties [7]. They adeptly account for variations in material composition and manufacturing processes.
This leads to research that is not only more tailored but also highly responsive to emerging data and trends. As a result, scientists can focus on experiments that deepen their understanding and align closely with their objectives.
Adaptable and Quick-Thinking Research Approach
This approach is about being flexible and fast in research, changing directions as needed based on new findings and assessments. It's a perfect mix of cutting-edge methods and expert insights. This synergy speeds up innovation by combining the best of technology and human expertise. It's a collaborative effort that not only conserves time, money, and resources but also significantly boosts the likelihood of major breakthroughs.
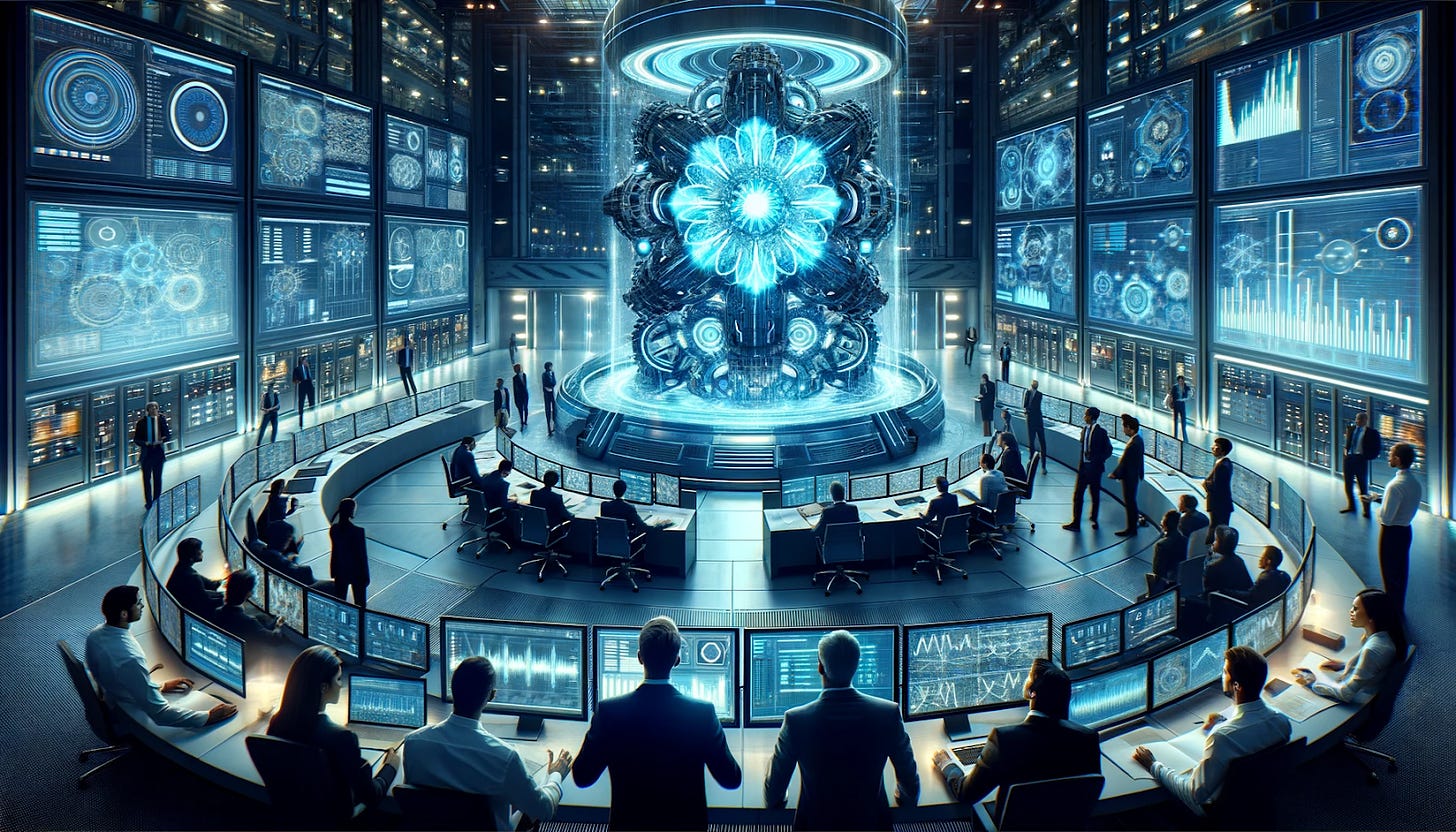
Section 3: Real-World Impact of Machine Learning and Adaptive Experimental Design in Battery Technology
The integration of machine learning with adaptive experimental design represents more than just theoretical advancement; it is revolutionising the development of batteries. This is yielding significant enhancements in efficiency, reliability, and sustainability of battery technology.
Enhance Electric Vehicle Battery Management
In the field of electric vehicles (EVs), the influence of machine learning is especially significant. It plays a vital role in precisely forecasting key battery metrics, such as state of charge and health, as illustrated in Fig. 3 [3]. These advancements not only prolong battery lifespan but also enhance the overall performance of EVs. This progress is crucial in addressing major concerns surrounding the adoption of electric vehicles.
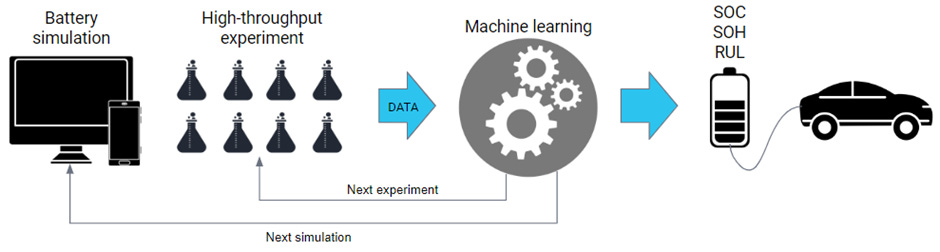
Lithium-Ion Battery Optimisation
Lithium-ion batteries, fundamental to contemporary portable and renewable energy storage, are experiencing a significant transformation. AI-driven strategies are refining electrode compositions and structures [8], resulting in batteries that boast higher energy densities and extended lifespans. These AI models play a crucial role in surpassing the limitations of conventional battery design, facilitating the development of batteries that are both more efficient and powerful.
Pioneer Battery Electrode Manufacturing
A significant breakthrough has been achieved in the manufacturing of Lithium-Ion Battery electrodes, especially in the automotive sector [9]. Researchers have merged physics-based simulations with machine learning to pinpoint the most optimal manufacturing parameters. This has led to enhancements in the texture and performance of the electrodes. This methodology not only elevates battery efficiency but also showcases cost-effectiveness and resource conservation, minimising waste.
Advanced Methods in Battery Health Monitoring
Machine learning-based techniques are revolutionising the field of battery health monitoring [10]. These advanced methods analyse data, such as discharge curves, to precisely track battery performance. They adapt to a range of conditions, so can be used to significantly extend the functional lifespan of batteries.
Wider Implications for Other Industries
This technological evolution transcends the battery industry, with machine learning and adaptive experimental design set to impact a wide array of sectors. From the automotive industry [3] to renewable energy systems [11], these advances have the potential to revolutionise various fields (Fig. 4). By enabling more efficient and sustainable practices, they are charting a course towards a future that is both technologically advanced and environmentally responsible [12]. In light of these developments, it is crucial to ponder the wider ramifications for other industries. How might they redefine our overall approach to energy and technology? Delving into these questions is key to grasping the extensive possibilities of these technological innovations.
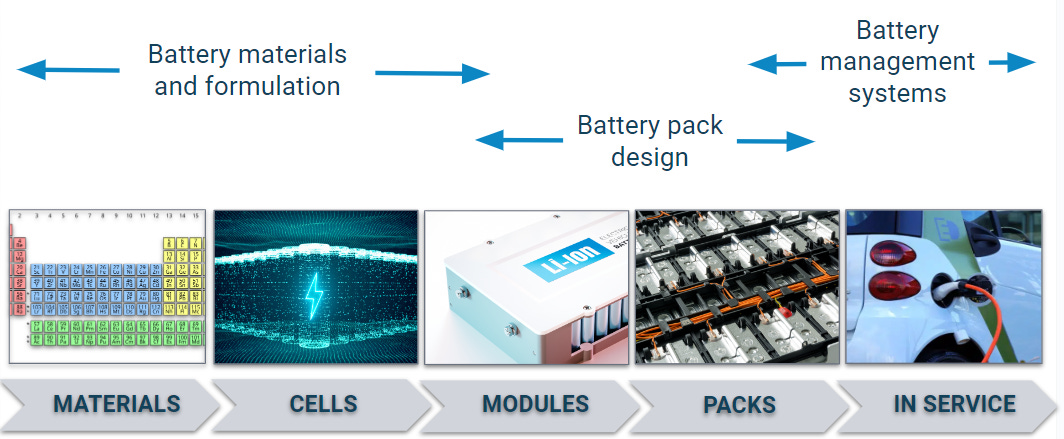
Conclusion: Paving the Way for an Electrified Future
The fusion of machine learning with adaptive experimental design is revolutionising battery technology, heralding a shift towards energy storage solutions that are more efficient, reliable, and sustainable. This transformation extends beyond merely speeding up innovation; it ensures that advances are not only swift but also practical, sustainable, and attuned to market needs.
Breakthroughs in battery technology promise to redefine energy storage in key areas like electric vehicles and renewable energy systems. The combination of AI and adaptive experimental design is a critical step to reduce the environmental impact of battery production, aligning with global sustainability goals [12]. The true value of this revolution lies in its contribution to a future that is both efficient and responsive to evolving market demands.
As we stand at the cusp of these significant advancements, it is vital to explore further possibilities. What new developments could arise from the amalgamation of AI and adaptive experimental design in energy storage and beyond? How might these technologies continue to transform the realms of energy, transportation, and environmental sustainability? Embarking on this electrified future, we must envision and prepare for the diverse opportunities that await.
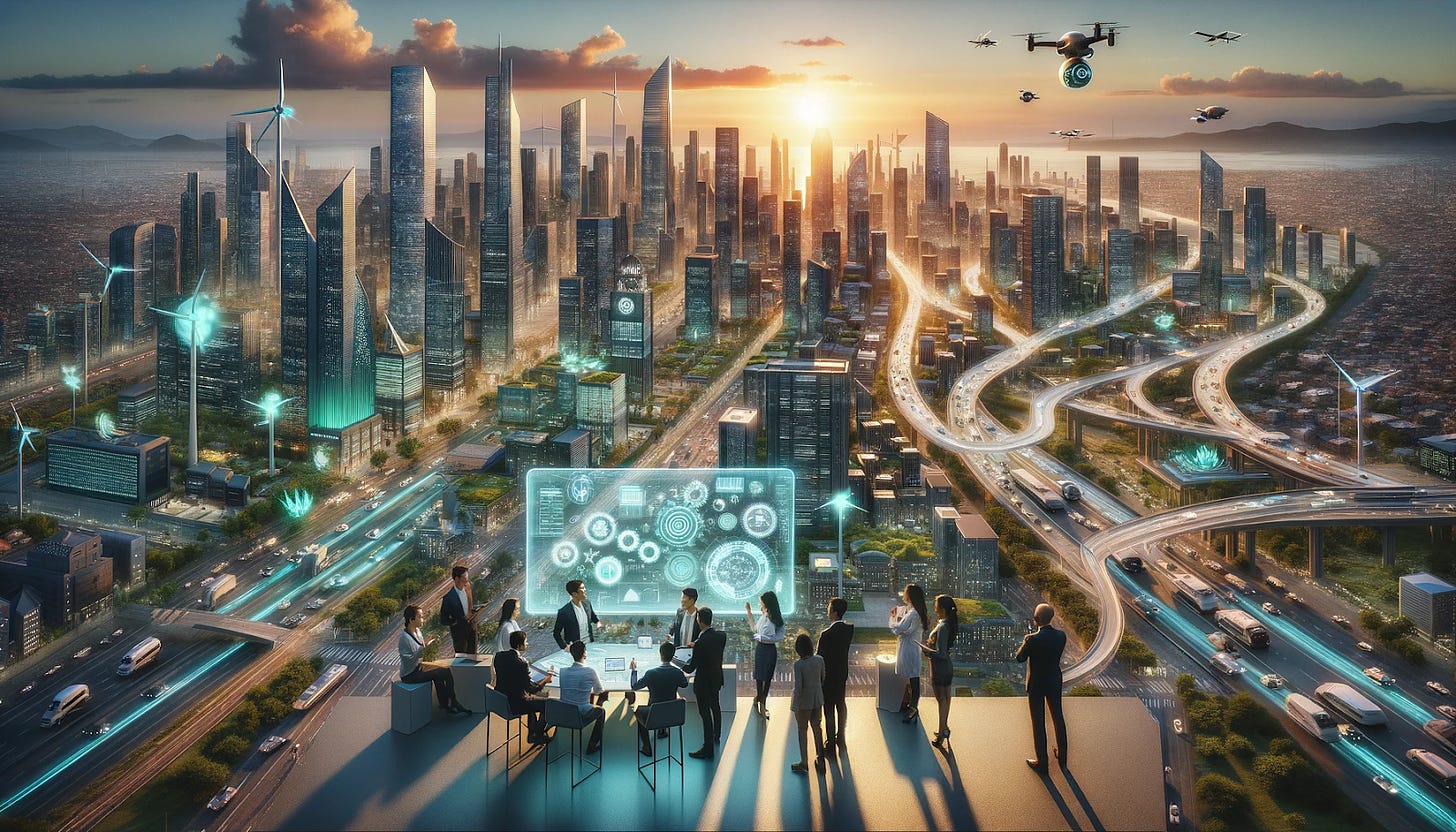
🌞 Thanks for reading!
📧 For tips, feedback, or inquiries - reach out
🌐 Follow us on Twitter, LinkedIn, and our website
References
Strickland J., Woolston P., Whitehead T. Adaptive Experimental Design (In Review)
Lombardo, Teo, et al. "Artificial intelligence applied to battery research: hype or reality?." Chemical reviews 122.12 (2021): 10899-10969.
Ng, Man-Fai, et al. "Predicting the state of charge and health of batteries using data-driven machine learning." Nature Machine Intelligence 2.3 (2020): 161-170.
Intellegens. "Case Studies." Accessed January 2, 2024. https://intellegens.com/article-type/case-studies/.
Verpoort, P. C., P. MacDonald, and Gareth John Conduit. "Materials data validation and imputation with an artificial neural network." Computational Materials Science 147 (2018): 176-185.
Conduit, B. D., et al. "Probabilistic design of a molybdenum-base alloy using a neural network." Scripta Materialia 146 (2018): 82-86.
Zviazhynski, Bahdan, and Gareth Conduit. "Unveil the unseen: Exploit information hidden in noise." Applied Intelligence 53.10 (2023): 11966-11978.
Drakopoulos, Stavros X., et al. "Formulation and manufacturing optimization of lithium-ion graphite-based electrodes via machine learning." Cell Reports Physical Science 2.12 (2021).
Duquesnoy, Marc, et al. "Machine learning-assisted multi-objective optimization of battery manufacturing from synthetic data generated by physics-based simulations." Energy Storage Materials 56 (2023): 50-61.
Sheikh, Shehzar Shahzad, et al. "A battery health monitoring method using machine learning: A data-driven approach." Energies 13.14 (2020): 3658.
Engineering Institute of Technology. (n.d.). Making Smart Grids Smarter with Machine Learning. Retrieved from https://www.eit.edu.au/making-smart-grids-smarter-with-machine-learning/.
David Sandalow, Colin McCormick, Alp Kucukelbir, Julio Friedmann, Trishna Nagrani, Zhiyuan Fan, Antoine Halff, Alexandre d’Aspremont, Ruben Glatt, Elena Méndez Leal, Kevin Karl and Alex Ruane, Artificial Intelligence for Climate Change Mitigation Roadmap (ICEF Innovation Roadmap Project, December 2023). Available at https://www.icef.go.jp/roadmap/