BattGPT or AI bubble?
Learning about AI and how they apply to batteries with Gaël Mourouga 🤖 If you enjoy this newsletter, give us a share and subscribe!
BattGPT or AI bubble?
You’ve probably heard of Stable Diffusion and ChatGPT, some of the AI models which have (rightfully) been making headlines recently. So naturally, we asked ourselves the question: what’s coming for batteries?
In this article, we:
Look at examples where AI was successful. So far, the successful fields for AI applications were those where input data was available in large quantities and relatively easy to interpret: games (chess, Go), image processing, text processing, among many others.
Look at possible bottlenecks in the application of AI to batteries. Batteries are complex systems, where data is not that easy to acquire in a strictly reproducible way, and knowledge of the underlying physics matters.
Assess the current state-of-the-art and where bottlenecks are easier to solve. We review startups from our database, looking at possible applications in materials discovery, manufacturing, battery modelling, and battery management systems.
Are we heading towards an AI bubble?
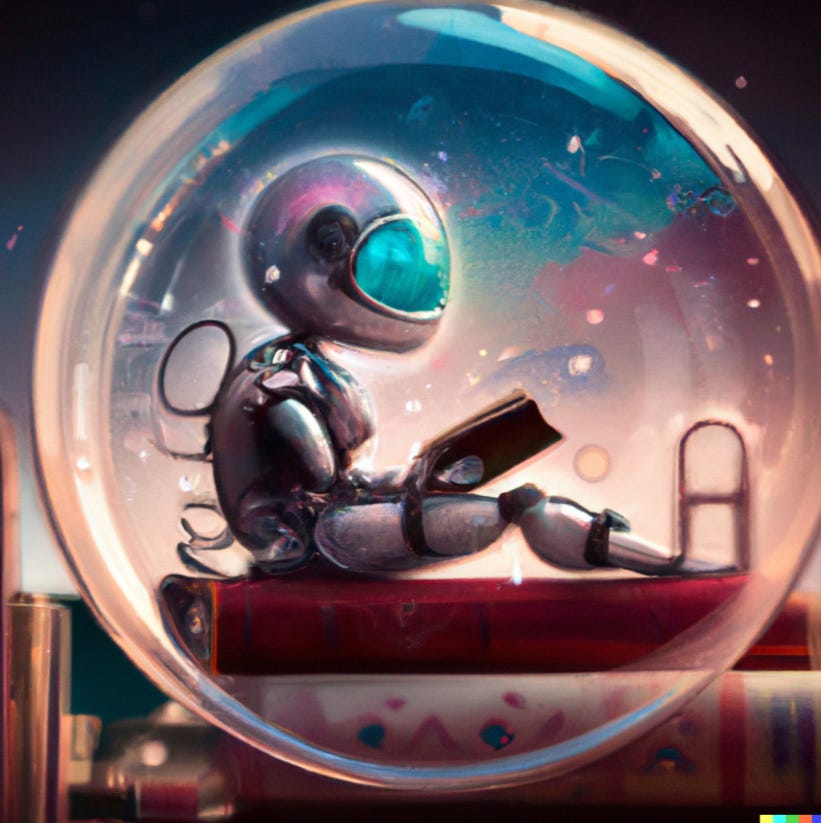
With incredible breakthroughs in generative AI (like Dall-E’s prompt-to-image capabilities, as shown above), there has been a massive coverage of this technology, and leading companies are rightfully gaining a lot of valuation. While the hype is deserved, it also leads to fears of an AI bubble, and parallels are already being drawn between the field of AI in 2023 and the 2000 dotcom bubble or the 2021 web3 frenzy. In short, there is a fear of an uptick in companies advertising “AI” in the hope of attracting VC funding, the same way companies were adding “dotcom” at the end of their name in the late 90s, or putting “blockchain” or “crypto” in front of whatever they were developing around 2020.
What chatGPT thinks
ChatGPT, if you haven’t heard of it, is a prompt-to-text model that answers questions formulated in natural language. Its capabilities in terms of comprehension and clarity of expression are nothing short of amazing, so I asked it, “how can AI be used to aid battery research and development?”:
Artificial intelligence can be used in various ways to aid battery research and development. Some potential applications include:
Data analysis
Predictive modeling
Materials discovery
Process optimization
Then, it described these applications in more detail, in its usual surprisingly-accurate-but-ultimately-quite-shallow three-sentence style that you are probably familiar with if you have tested it already.
I couldn’t help but notice a lot of similarities with the conclusion of this article from Lombardo et al., recently published in Chemical Reviews: Artificial Intelligence Applied to Battery Research: Hype or Reality? I met Teo Lombardo during my academic visits to Amiens, France. He was a PhD student in the ERC-funded ARTISTIC project working on 3D physics-based models of Li-ion battery electrode manufacturing. He is currently working in the research group of Prof. Jürgen Janek (Germany) on the coupling between experimental analytics and data science. We had a chat on batteries and AI, trying to go deeper into what chatGPT had sketched for us.
Data is king
Data was one of our main talking points. Broadly speaking, machine learning algorithms are fitting a huge number of weights and biases on a training data set before measuring the accuracy of its prediction on validation/test data sets.
Therefore, it is no surprise that data is at the centre of any AI framework, which becomes pretty obvious when you look at the most successful applications of AI (so far). I will stray a bit away from batteries in the following section to illustrate this point.
1. Games
The first “mainstream” application of AI which made headlines in 1997 was the victory of the supercomputer Deep Blue over chess world champion Garry Kasparov. Later developments of AI to competitive games would lead to the language-processing model Watson beating Jeopardy! contestants in 2011, and the artificial neural network AlphaGo beating Go world champion Lee Sedol in 2016. My point here is not how to explain how these algorithms work, but rather to look at the sort of data they were trained on, which would look like this for a game of chess:
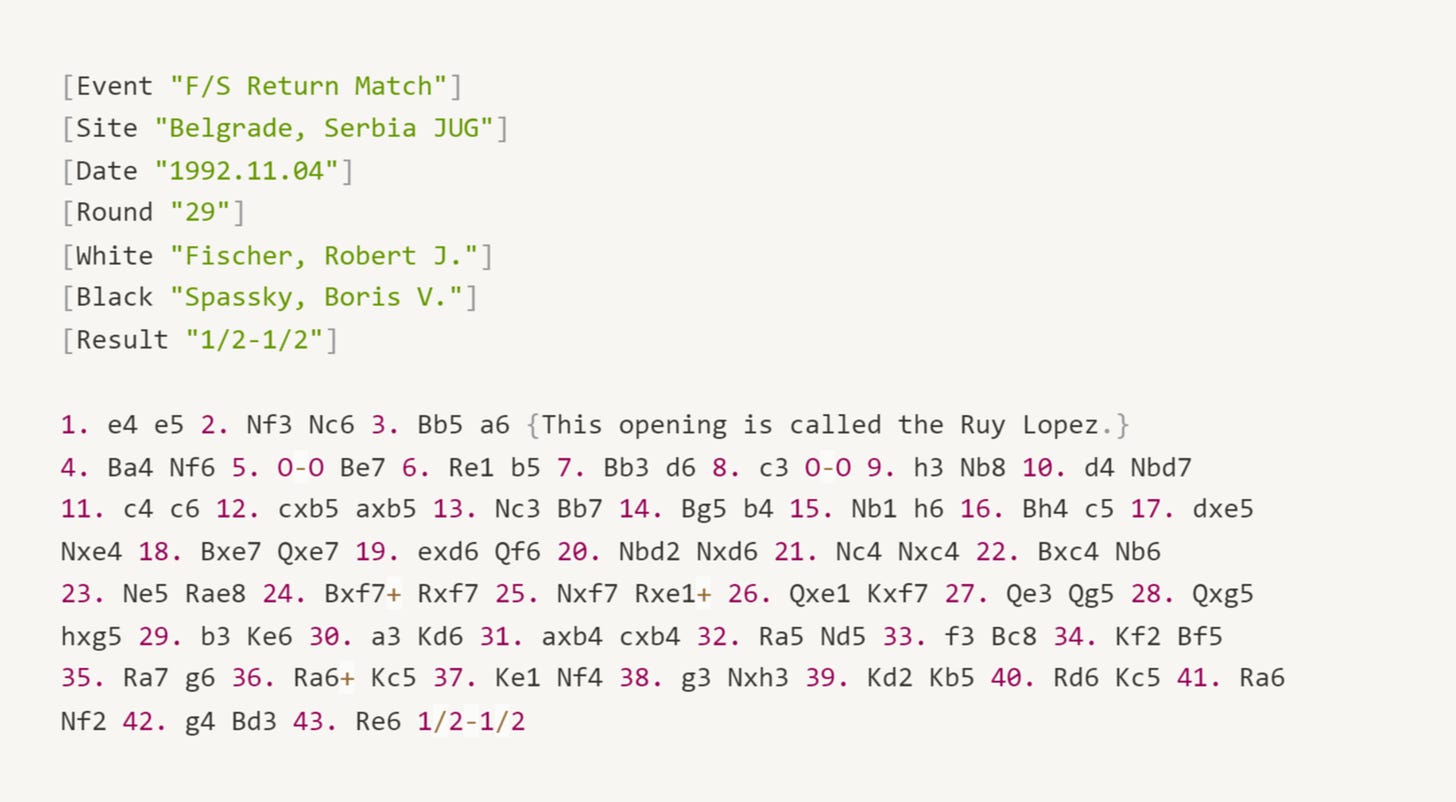
Websites like Chessgames.com host hundreds of thousands of chess games stored in similar formats, making for an efficient data pipeline to train AI algorithms.
2. Image recognition
I will leave it to the video from 3Blue1Brown on YouTube to explain how neural networks can recognize images, such as handwritten numbers.
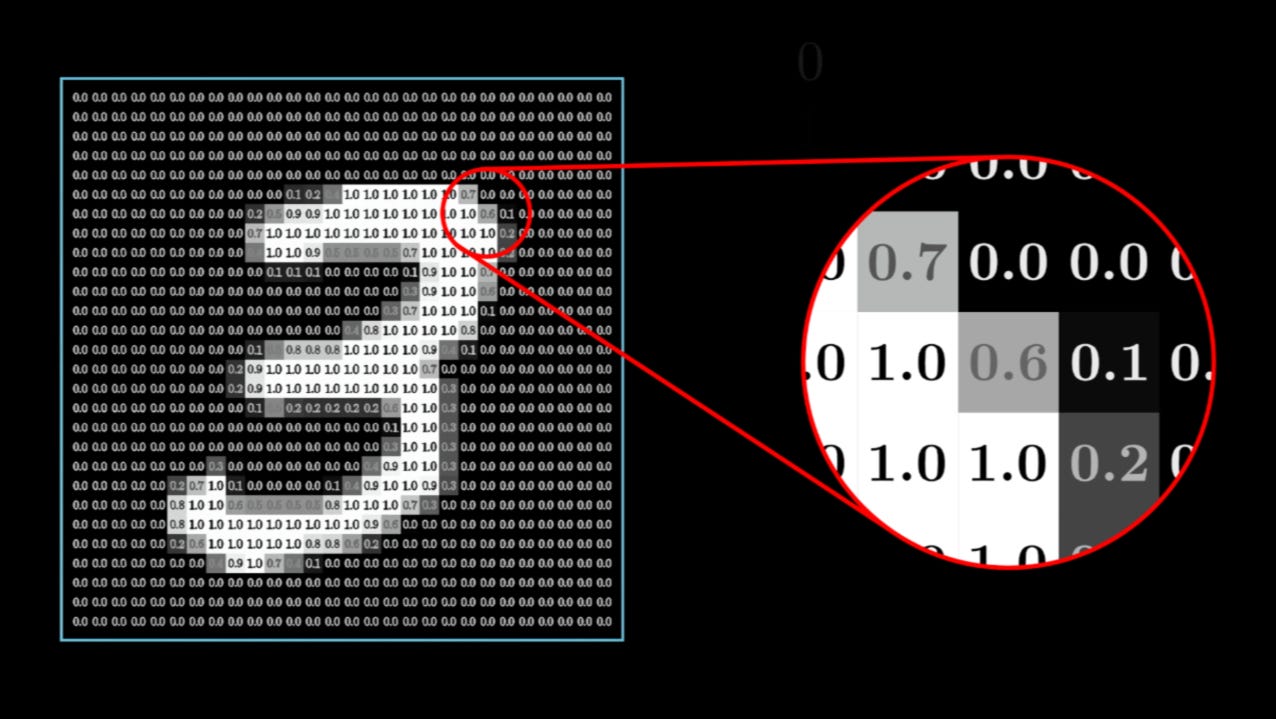
The leap forward we’ve seen in AI image recognition technologies (such as face recognition, fraud detection, healthcare, and many more) was made possible in no small part by the popularization of the Internet, smartphones and social media. Many images (from cats napping to humans partying) were suddenly available online, allowing researchers to feed them to neural networks and develop their image recognition capabilities. Some websites, such as ImageNet, were even created with the explicit purpose of providing tagged training, validation and test datasets for image recognition.
3. Language processing
You may be familiar with the translation software DeepL. It’s a deep neural network based on translated text data mined from the Internet using web crawlers. Their training datasets are composed of many millions of translated texts, where the network fits billions of parameters to a black-box language model.
Most of our direct competitors are major tech companies, which have a history of many years developing web crawlers. They therefore have a distinct advantage in the amount of training data available. We, on the other hand, place great emphasis on the targeted acquisition of special training data that helps our network to achieve higher translation quality. For this purpose, we have developed, among other things, special crawlers that automatically find translations on the internet and assess their quality.
For DeepL, quality (as well as quantity, in the sense that they still need millions of texts) of training data is key in developing performant AI algorithms.
Now, back to batteries.
Battery Data: a possible bottleneck?
Since battery data is the bread and butter of AI development, a good place to start might be the wealth of academic data contained in peer-reviewed publications, as mentioned in the introduction from the paper of Lombardo et al.
there is already a massive amount of data spread out in scientific publications: almost 30,000 LIB publications already exist, and this number is growing rapidly. A researcher reading 200 papers per year will need nearly 150 years to read all of the LIB publications available today.
There are, however, some problems with this data.
Uncertainties
Compared to the datasets I illustrated in my previous examples, battery data contains a lot more aleatoric and epistemic uncertainties. Small variations in things that can’t be controlled, like local electrode composition, experimental equipment deviation, or sensor drift, will affect results from one experiment to the other, even if the protocols are identical.
This lead to input data usually being presented in the form of a range of values (which can obey a certain distribution) rather than an exact value (i.e. a number on a grid or a letter in a text). For AI model developers, this poses the wider question of how to deal with uncertainty propagation.
Missing data (or worst)
People who have spent time in the lab will be familiar with Kahn’s second law: “An experiment is reproducible until another laboratory tries to repeat it”, backed up by data in this 2016 Nature article “1,500 scientists lift the lid on reproducibility” where up to 88% of the polled scientists in chemistry report difficulties in reproducing results from other researchers.
This can be attributed to several reasons: from p-hacking (selecting the best results for publishing and under-reporting the real uncertainty range) to hiding details from the experimental protocol.
An example of the latter I encountered during my academic visits: performing membrane conductivity measurements in a non-thermostated lab, where “room temperature” can reach a comfortable 40°C / 104°F in summer. While it is technically “at room temperature,” a researcher looking to reproduce these results (or an AI algorithm using the data) may guess a more standard 25°C / 77°F.
Even without intentionally missing details in a protocol, there is generally an absence of standards in reporting data in the battery literature, which can be problematic for data gathering. Lombardo et al. cite the work of El-Bousiydy et al., who applies text mining to the battery literature, specifically looking for information on electrode and electrolyte composition or cycling conditions:
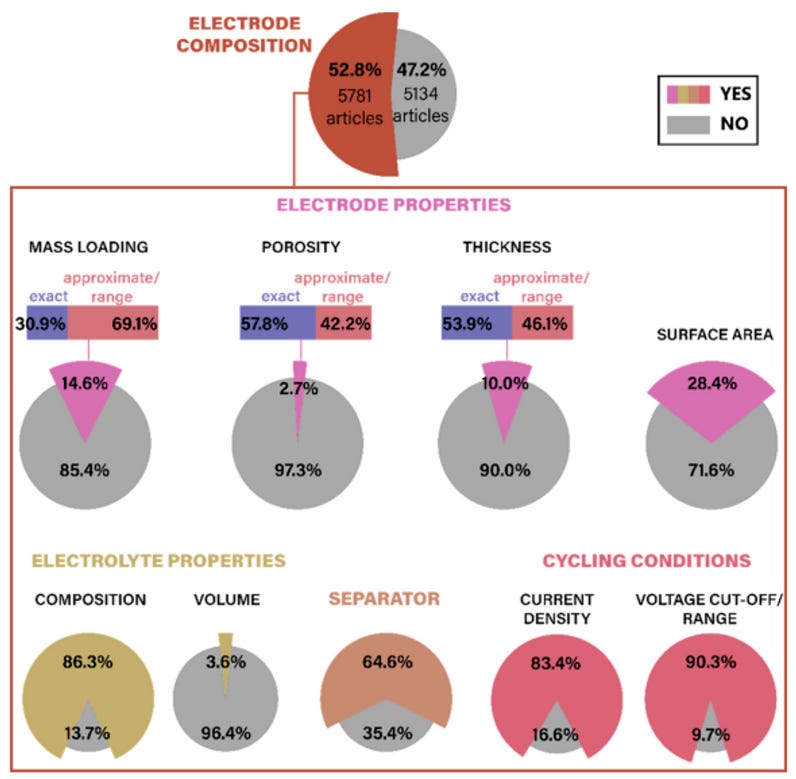
The amount of missing and/or inaccurate data can be problematic to correctly interpret the results of different papers, and ultimately to train AI algorithms. It calls for better defined standards for reporting data in the battery community.
Publish or Perish
Another problem is inspired from the excellent, somewhat satirical, paper from Wang et al. “Will any crap we put in Graphene enhance its electrocatalytical effects?”:
One may exaggerate only a little by saying that if we spit on graphene it becomes a better electrocatalyst. Having 84 reasonably stable elements (apart from noble gases and carbon), one can produce 84 articles on monoelemental doping of graphene; with two dopants we have 3486 possible combinations, with three dopants we can publish 95,284 combinations, and with four elements there are close to 2×10^6 combinations.
The authors go on to demonstrate that guano (the scientific name for “bird s**t”) increases the electrocatalytic effects of graphene by a non-insignificant margin. This poses the question of how reliable literature datasets are (even in the absence of p-hacking or missing data) since researchers have an incentive to publish as much as possible. What if this results in artificially inflated experimental spaces to explore?
Industry: any better?
Therefore, one may rather look at the industry to provide curated, high-quality experimental data to train machine learning algorithms on. As Lombardo et al. mention in their introduction
BASF, the second largest chemical producer in the world, recently announced that they produce >70 million battery characterization data points per day
The first question here is that of incentives. It is unlikely that major battery manufacturing companies (LG Chem, CATL, NorthVolt, etc.) or auto OEMs (Tesla, BMW, …) will spontaneously make all of their data accessible online, open-source. And if they do, they might become subject to the same biases as academics. Then, there’s the question of incentives for the code developers: GitHub Copilot, a Microsoft code autocomplete program for developers, uses open-source code stored on the GitHub platform to train itself. This generated a backlash in the open-source community, as the GitHub Copilot code itself was kept closed-source.
Some initiatives exist to provide standardized battery data, like the Battery Index or the ARTISTIC database, but these projects are still in their infancy. And, even with years of development, it is unclear whether they can provide enough high-quality battery data to train AI algorithms, the way ImageNet did for image recognition or go4go.net did for the game of Go.
Physics: another possible bottleneck?
So far, I’ve only talked about pure data-based machine learning, i.e. algorithms where the model is discovered from scratch by a sort of numerical trial-and-error. However, physics-informed machine learning also exists, where equations are integrated to the machine learning algorithm. I will leave this excellent introductory YouTube video from Steve Brunton on the topic, from which I particularly liked the example of Heliocentrism versus Geocentrism:
Suppose you trained a machine learning algorithm on planets’ trajectory data. Which model would it come up with? Geocentric or heliocentric ? Which one is “better”? It’s very likely that if you don’t feed the algorithm any physics, it would fit a bunch of Fourier transforms (like Ptolemy did) to measurement data, which are accurate but ultimately lack a profound physical understanding of the system under study.
The physics of self-driving cars will be cracked… next year
Self-driving cars is an interesting field. Despite years of development and lots of investment in the field of machine learning and AI to help with decision-making, it has so far failed to deliver the same kind of mainstream milestone as AlphaGo beating world champion Lee Sedol, or AlphaFold solving protein folding.
Why is that the case, despite EV operators having already gathered a huge amount of sensor data over the last decade? That’s partly because a lot of different sensors are required. Image recognition is only a very small part of the problem: algorithms also need to learn how to orientate the car in space, predict trajectories of moving objects (including other cars driven by humans), anticipate things such as the impact of rain, deal with limited information on their surroundings, account for measurement uncertainties, etc.
Teo and I are nevertheless hopeful about the future of self-driving cars, with companies like Cruise (General Motors), Waymo (Google) and Zoox (Amazon) potentially driving the field forward in the near future. I also firmly believe that advancements in this field will lead to spillovers in battery research, given how adjacent the two fields are and how similar their problems are.
I will recommend this excellent article published by our friends at BatteryBits from Siyi Zhang, “Lessons Learned as an AI Product Manager at Tesla Energy”, where she expertly breaks down potential pitfalls in the application of AI to energy applications from her experience at Tesla (not just in self-driving).
The article is from 2021, and a lot has happened since in both AI and the energy sector. Let me add my analysis and predictions related to the battery market.
The current state of AI in the battery industry and some predictions
To assess the state of the market, I reviewed 13 companies’ websites mentioning both batteries and AI, taken from our internal database. I looked at the information on the websites (links to peer-reviewed publications, team members on LinkedIn, etc.) and conducted a patent search. I then evaluated these companies based on three criteria: